The research titled “Inter-City Firm Connections and the Scaling of Urban Economic Indicators” by Yang, Jackson, and Kempes, published in PNAS Nexus (Nov 2024), presents a fresh perspective on how cities generate economic output. While traditional urban scaling theories focus on how local, intra-city interactions drive economic productivity, this study argues that inter-city connections — especially through multinational firms — play an equally, if not more, significant role. By analysing GDP data from cities in the US, EU, and PRC, alongside the Global Network Connectivity (GNC) of multinational firms, the study reveals that cities with higher inter-city connectivity exhibit higher-than-expected GDP, even after accounting for population size. This finding challenges the conventional idea that urban scaling is driven solely by local social interactions, offering a new lens for understanding complexity in urban systems.
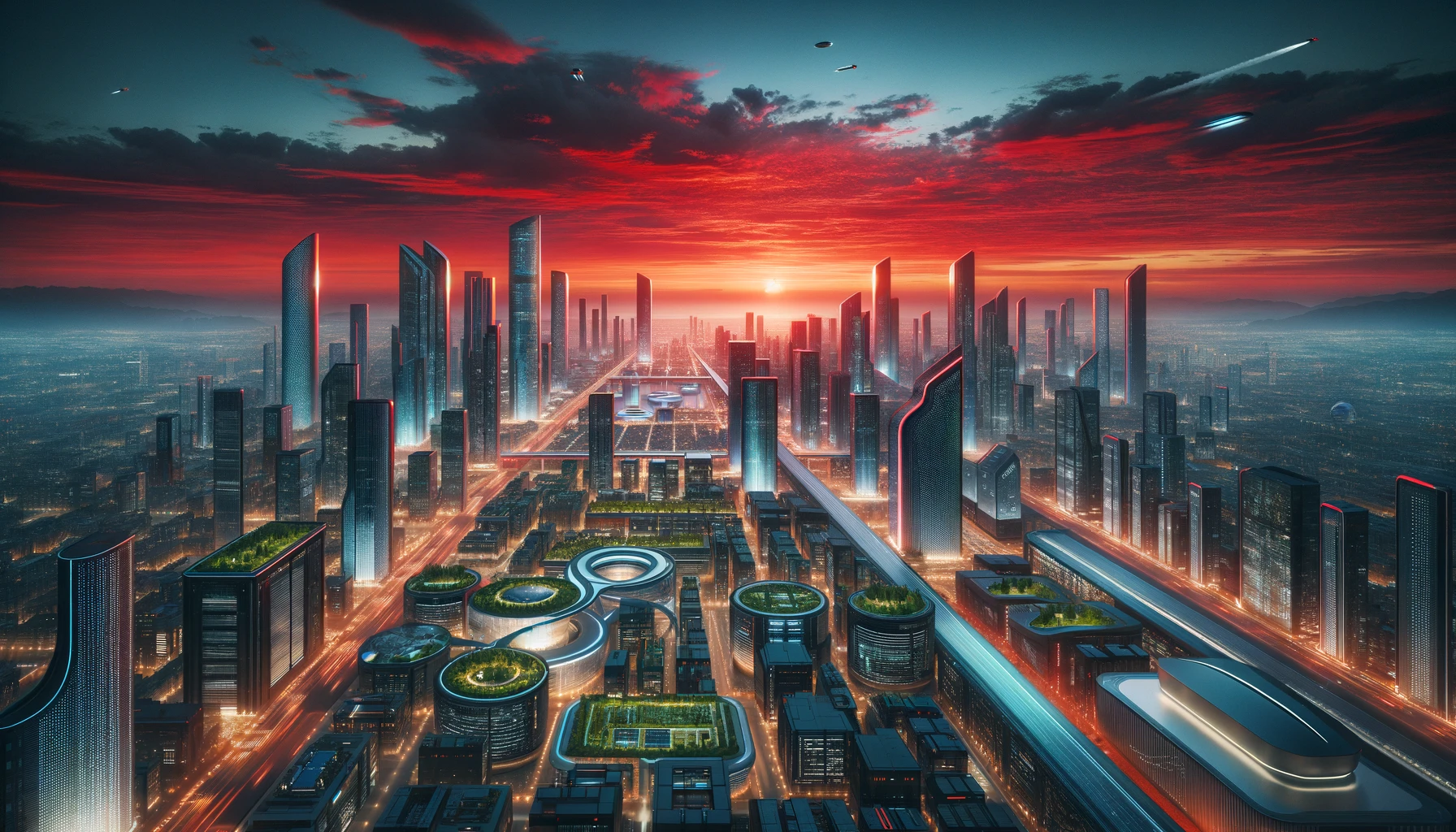
This study is an example of how complexity science can be applied to real-world systems like cities. Cities, as complex adaptive systems (CAS), exhibit emergent behaviours, such as superlinear scaling of GDP, where larger cities tend to be disproportionately more productive. Traditionally, this emergent property was attributed to denser local social interactions. However, the authors introduce a new dimension of complexity by demonstrating how inter-city firm connections serve as an additional mechanism for economic emergence. Using the concept of networked systems, cities are modelled as nodes connected by firms, and the GNC score quantifies the strength of these connections. The research shows that GDP is influenced not just by a city’s local population but also by its position within this global network. This insight extends the complexity science framework by highlighting the role of cross-city organisational linkages in shaping global economic output.
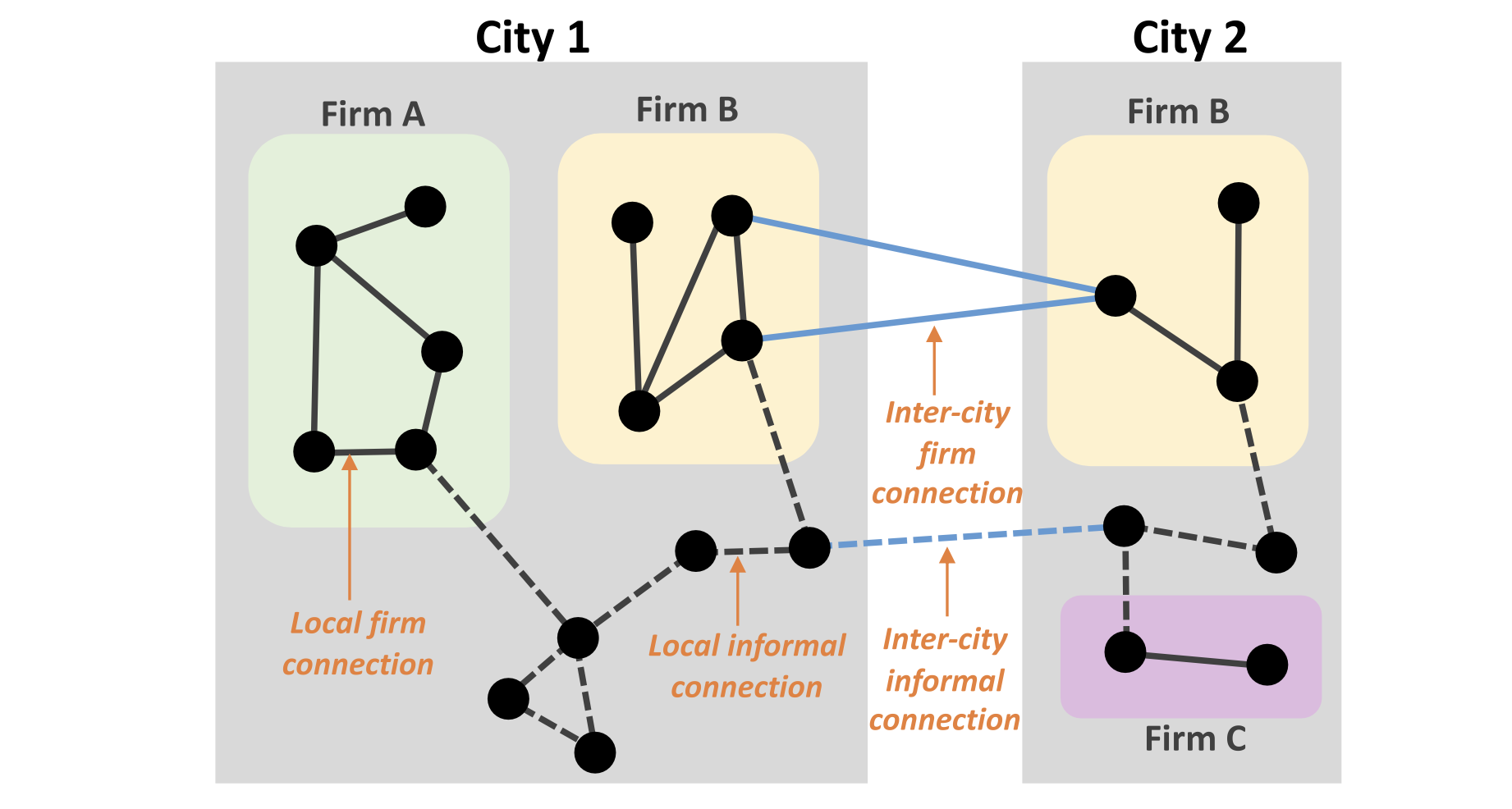
The study also provides methodological advances that enrich the complexity science toolkit. It uses Scale-Adjusted Metropolitan Indicators (SAMI) to compare how cities “overperform” or “underperform” in GDP relative to expectations. This allows for a nuanced view of which cities benefit most from inter-city connections. Furthermore, the use of multilevel regression models that incorporate both local (population) and global (GNC) factors reveals the nonlinear dynamics at play. Such nonlinear scaling, where population alone cannot explain GDP growth, suggests the presence of feedback loops where better-connected cities become more prosperous, and prosperous cities become better connected. These insights underscore how complexity science can offer more accurate, multi-layered models of urban growth, moving beyond simplistic population-based approaches.
The implications of this research go beyond academic curiosity. For policymakers, it suggests that urban economic development strategies should prioritise enhancing global connectivity. Cities can benefit from strengthening ties with multinational firms, facilitating cross-city collaborations, and becoming key nodes in the global urban network. This is a shift from the classic focus on improving only local conditions, such as infrastructure or intra-city mobility. For complexity science, this study exemplifies how theories of self-organisation, emergence, and adaptive networks can be operationalised in practical, high-impact research. The work highlights the potential for developing a more comprehensive urban scaling model that integrates both local and global processes. By bridging concepts from complexity science with urban development, the study opens new possibilities for future research into how global interconnections influence local outcomes, from economic growth to social inequality.
Source: Vicky Chuqiao Yang, Jacob J Jackson, Christopher P Kempes, 2024, Inter-city firm connections and the scaling of urban economic indicators, PNAS Nexus 3:11, DOI: 10.1093/pnasnexus/pgae503